|
- An integrated model for cellular analysis
- Eduardo Battistella, José G.C. de Souza, Cláudia K. Barcellos, Ney Lemke and
- José C.M. Mombach
- Laboratório de Bioinformática e Biologia Computacional, Universidade do Vale do Rio dos Sinos, Unisinos, Av. Unisinos, 950, Caixa Postal 270, 93022-000 São Leopoldo, RS, Brasil
- Corresponding author: J.G.C. de Souza
- E-mail: [email protected]
- Genet. Mol. Res. 4 (3): 506-513 (2005)
- Received May 20, 2005
- Accepted July 8, 2005
- Published September 30, 2005
ABSTRACT. We present the MOlecular NETwork (MONET) ontology as a model to integrate data from different networks that govern cell function. To achieve this, different existing ontologies were analyzed and an integrated ontology was built in a way to make it possible to share and reuse knowledge, support interoperability between systems, and also allow the formulation of hypotheses through inferences. By studying the cell as an entity of a myriad of elements and networks of interactions, we aim to offer a means to understand the large-scale characteristics responsible for the behavior of the cell and to enable new biological insights.
Key words: Ontology, Cellular function, Knowledge data discovery, Data integration
INTRODUCTION
One of the most important challenges for biology in the postgenomic era is to understand the structure and behavior of the complex intercellular Web of molecular interactions that control cell behavior (Barabási and Oltvai, 2004). The basis to achieve this goal has already been built.
There are more than 548 biological data sources available on the Internet (Bateman, 2004). They hold data, such as genomes, mRNA, protein structures, protein-protein interactions, cellular signaling, metabolic pathways, and transcription-regulatory networks. This huge and complex set of data collected during recent years harbors information that requires an integrative approach (Uetz et al., 2002). Computer scientists and biologists will need to use innovative methodologies to deal with them.
The key to understand the structure and behavior of the cell is to integrate the available data in a way that it increases our understanding of the underlying biological processes that operate inside the cell (Ideker et al., 2001; Uetz et al., 2002; Barabási and Oltvai, 2004; Yeger-Lotem et al., 2004). Integrated biological models that assimilate this knowledge are essential to formulate new hypotheses, to predict cellular behaviors that can be tested experimentally (Ideker et al., 2001), and for a complete understanding of the cell. But the integration task is not simple.
Biological data are disseminated in many different databases. These databases have different management systems, formats and views of how to represent the data stored. Most of them are accessible by flat files or by web interfaces that allow some kind of query. The two main problems are the difficulty in parsing the data when dealing with heterogeneous flat file formats and the inconsistency due to the absence of a unified vocabulary, which means that the same information is represented in more than one way. Fortunately, ways to improve this scenario already exist.
Ontologies are an important approach to bring order to this scenario and to enable an integrated view of these data. An ontology is an explicit specification of a conceptualization (Gruber, 1993). While controlled vocabularies (e.g., Resource Description Framework (RDF), Extensible Markup Language (XML) Schema) only restrict the words used to describe a domain, ontologies extend this simple control vocabulary feature and allow the formal specification of the terms and the relations among them. They make sharing and reusing the knowledge possible, support the interoperability between systems, and also allow inferences from them. In bioinformatics, ontologies are crucial for maintaining the coherence of a large collection of complex concepts and their relationships (Backer et al., 1999).
In this context, we present the MOlecular NETwork (MONET) ontology. MONET ontology is a proposal to integrate data from the “network of networks” (Barabási and Oltvai, 2004) that exist inside the cell, helping us to understand the large-scale characteristics responsible for the behavior of the cell and enabling new biological insights. In short, it provides a way to cross the bridge between data and knowledge.
DOMAIN ANALYSIS
Bioinformatics is a growing field for ontologies (Battistella et al., 2004). As in other hot-spot areas, new ontologies are frequently proposed, but the “infant mortality” is high. We present some of the ontologies available for the molecular biology domain. We opted for the ones that have shown a continuous investment in research, resulting in new features/tools, and those whose proposals seem to have a promising future and could be adopted broadly.
One of the most ambitious projects of ontology applied to biology is the Gene Ontology Consortium (GO)
(http://www.geneontology.org). GO aims to provide an ontology that covers several domains of molecular and cellular biology (Gene Ontology Consortium, 2004). It is structured into three sub-ontologies: biological processes (formed by one or more assemblies of molecular functions), molecular function (describes activities at a molecular level), and cellular component (enumerates the locations in a cell, considering subcellular structures). These sub-ontologies have been built to be used in the annotation of genes, gene products and sequences.
The Sequence Ontology Project (SO)
(http://song.sourceforge.net) is a joint effort by genome annotation centers (WormBase, the Berkeley Drosophila Genome Project, FlyBase, the Mouse Genome Informatics group, and the Sanger Institute) that aim to offer an ontology suitable for sequence annotation and for data exchange of this annotation. It is under development, and its interim releases are made available as soon as they are considered to be usable. Examples of concepts available at SO are: intron, exon, gene, polypeptide, protein, DNA, RNA, mRNA, tRNA, and rRNA.
The Proteomics Standards Initiative (PSI) Molecular Interaction (MI)
(http://psidev.sourceforge.net) ontology aims to represent interactions among proteins. PSI MI, an effort of the Human Proteome Organization (HUPO), was implemented through a specification of an ontology and an XML Schema. Both are being developed with a multi-level approach (Orchard et al., 2003; Hermjakob et al., 2004). The current level implements declarative representations of molecular interaction concepts divided into: interaction type, sequence feature type, feature detection, participant detection, and interaction detection. The interaction type vocabulary describes the type of connection found between molecules. The sequence feature type describes the relevant properties for the binding of proteins. The other three vocabularies describe the method by which the feature was detected.
The primary purpose of the Microarray Gene Expression Data (MAGE)
(http://www.mged.org) ontology is to provide standard terms for the annotation of microarray experiments. Microarray data require complex structures, making some processes difficult, such as data-interchange and data documentation (Spellman et al., 2002). There have been various types of representations for microarray data, which make the reproduction of experiments a problematic task (Brazma et al., 2001). This ontology, which is currently under development, enables unambiguous descriptions of how the experiment was performed.
Other ontologies can be found at Open Biological Ontologies (OBO)
(http://obo.sourceforge.net). OBO is an effort focused on the production of research that intends to facilitate the sharing of ontologies from different biological domains. All ontologies are open for use by the scientific community, and they are a useful starting point for new ones.
The ontologies presented here are not the only ones. There are other proposals, such as Transparent Access to Multiple Bioinformatics Information Sources (Goble et al., 2001), and proprietary ones, such as EcoCyc (Karp, 2000) ontology. New efforts are being launched, such as BioBabel (a new European project being coordinated by the European Bioinformatics Institute - EBI -
http://www.ebi.ac.uk/biobabel). BioBabel aims to enhance the data interchange of biological databases by standardization of biochemical terminology.
All these ontologies show how the efforts to cover the vast area of molecular biology were developed until now. Based on these ontologies, and because of the need for an integrated approach, we introduce MONET.
MONET ONTOLOGY
There is a need for ontology proposals that allow an understanding of how the molecular networks inside a cell determine cell behavior (Ideker et al., 2001; Uetz et al., 2002; Barabási and Oltvai, 2004; Yeger-Lotem et al., 2004). Among other requirements, the proposal must be able to minimize data redundancies and inconsistencies. The data-interchange problem must be taken into consideration through the adoption of free and open standards. It also needs to be extensible, so new knowledge can be easily implemented by the aggregation of new concepts.
MONET integrates information from transcription-regulatory, metabolic pathway, and protein-protein interaction networks through a strategy that aims to establish a model able to minimize data redundancies and data inconsistencies. It is expandable, so new knowledge can be easily implemented. Even whole ontologies can be incorporated into MONET, which allows unlimited possibilities concerning the coverage of domains. Consequently, MONET allows the construction of topological models of cells of microorganisms, and the extension of these models becomes available as new knowledge.
The definition of an ontology is time consuming. An editor can result in a significant productivity profit. Among the available ontology editors we chose Protégé-2000
(http://protege.stanford.edu). The two main reasons for choosing Protégé were: a) the need, not only for a ontology editor, but for a Knowledge Base Management System, since we want to populate the database with examples from various microorganisms, and b) its open-source Java extensible architecture allows improvements in its functionality through the aggregation of new plugins. This latter characteristic allows the ontology to be exported in the different formats required by different research groups. A variety of import/export plugins can be used to automatically read/write the ontology in different representation data standards, such as Web Ontology Language (OWL), RDF, XML, and XML Schema.
The technical vocabulary used to describe MONET, concerning the ontology (not the biological knowledge), is based on Protégé. Its frame-based representation defines an ontology as a formal explicit description of concepts in a domain of discourse (concepts or classes), the properties of each concept describing various features, the attributes of the concept (slots or properties), and restrictions on slots (facets).
Figure 1 is a schematic representation of the main concepts implemented to achieve this integrated approach. Various types of concepts related to chemical molecules, such as DNA, RNA, mRNA, rRNA, tRNA, snRNA, and small metabolites, were omitted for simplification. We also omitted the slots of all concepts.
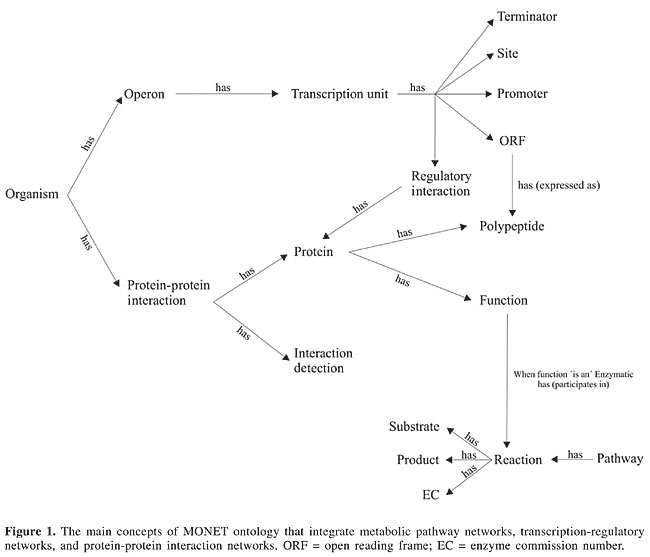
The transcription-regulatory network implements concepts, including operon (a set of genes transcribed under the control of an operator gene), transcription unit (part of DNA that will be transcribed into an RNA), terminator (DNA region where the transcription supposedly stops), ORF (a portion of a gene sequence that potentially encodes a protein), site (DNA sequence whose location and base sequence are known), promoter (a segment of DNA which provides a site where the enzymes involved in the transcription process can bind to a DNA molecule, and initiate transcription), and regulatory interaction (general information concerning the transcription-regulatory data being mapped).
The transcription-regulatory network is involved with interactions between DNA and proteins, and with the consequent production of proteins. The metabolic pathway network also involves proteins characterized by their enzymatic function. Proteins are the link between these networks.
The protein-protein interaction network has pairs of proteins whose interaction was detected experimentally or by an in silico process. This knowledge was also mapped into MONET. For each protein-protein interaction, we adopted from PSI MI ontology the concept of interaction detection (id MI:0001) and its subtree of concepts. The method to determine the interaction was divided into the sub-methods experimental and in silico, each with their corresponding possible notations.
The small molecule metabolism (metabolic pathway network) of MONET is a subset of the complete metabolism that excludes DNA replication and protein synthesis reaction. Beyond the concepts of reaction, substrate and EC (the enzyme commission number), other concepts, such as inhibitor, activator, kinetic, and chemicals, are involved. Although the structures of metabolic pathway networks and protein interaction networks are similar, there are a number of significant differences. While metabolic pathways focus on the conversion of small molecules and on the enzymes responsible for these conversions, protein interaction maps concentrate mainly on physical contacts, without obvious chemical conversions (Uetz et al., 2002).
The spatial aspect was also taken into consideration. MONET implements a concept entitled compartment to indicate the protein’s subcellular location. Consideration of the location of a protein and other chemicals is an important feature that allows more precise conclusions.
DISCUSSION
In our view, this model is a way to understand the internal organization and evolution of cells. It is not static, nor is it complete. But it is an important step in a direction that can lead us to a comprehensive modeling of the various networks that control the behavior of the cell.
The current version of this model implements metabolic pathway, transcription-regulatory, and protein-protein interaction networks. This model is being improved through the incorporation of a cell-signaling network.
MONET is neither better nor worse than GO, PSI MI, MAGE, SO, or other ontologies. It has a different point of view of how to model the knowledge. GO attacks the annotation problem; MONET is not in this stage yet. PSI MI deals with molecular interactions; MONET also deals with this problem, and it incorporates most of the concepts available in PSI MI. MAGE covers microarray experiments; MONET does not. SO offers sequence annotation and provides for data interchange of this annotation; MONET also does so by incorporating most SO concepts.
While these other ontologies are specific to a particular aspect of the molecular biology domain, MONET extends them and integrates them as a whole, giving a holistic view of the cell, allowing for “functional bioinformatics” (Karp, 2000). This bioinformatics makes the development of new algorithms, graphical visualization interface, and many other tools that aid in the investigation of the principles that govern cellular function, possible.
We intend to populate our knowledge base with information from some microorganisms. We already started this process with the incorporation of the KEGGs Ligand database
(http://www.genome.ad.jp/kegg) as part of the metabolic pathway networks. This was not a simple task. To achieve this, we developed Python scripts to normalize the data available in the flat files, executed a series of consistency checks to correct the inconsistencies, and automated the generation of the instance flat file of Protégé (pins file). This process resulted in 21,430 small metabolites, 6,135 reactions, 4,327 enzymes, and 120 metabolic pathways.
We have also populated the knowledge base with protein and metabolic data from the microorganism Ureaplasma urealyticum. By doing so, we were able to export the metabolic data in XML format and load it in Mathematica 5.0
(http://www.wolfram.com) software, which allowed us to build up the metabolic network (Figure 2).

CONCLUSION
We present the MONET ontology as an integrated approach to build, test, and refine a model of the cellular pathway of organisms. It remains a challenge to integrate data from the myriad interactions of the cellular constituents. One may contest our view of how to model these networks and to integrate them. This is one of the possible variations that concern this complex, constantly changing, and not yet completely understood, area of molecular biology.
The future will bring new graphical interfaces to visualize and to analyze these networks, and will also bring new integrative models on which simulations may be performed, fundamentally improving our view of cell biology.
The next steps in our work are to refine MONET, including concepts such as cellular signaling, and to use this ontology to build a knowledge base for the microorganisms Escherichia coli, Helicobacter pylori and Mycoplasma pneumonia. We expect to simplify and speed up the extraction of relevant biological knowledge with this topological integrated model of an organism.
Copies of the MONET ontology (in Protégé, OWL, RDF, and XML Schema formats) are available upon request from the authors.
ACKNOWLEDGMENTS
Research supported by FAPERGS and CNPq, process number 401999/2003-3. This work was developed in collaboration with HP Brazil R&D.
REFERENCES
Baker, P.G., Goble, C.A., Bechhofer, S., Paton, N.W., Stevens, R. and Brass, A. (1999). An ontology for bioinformatics applications. Bioinformatics 15: 510-520.
Barabási, A.-L. and Oltvai, Z.N. (2004). Network biology: Understanding the cell’s functional organization. Nat. Rev. Genet. 5: 101-113.
Bateman, A., Coin, L., Durbin, R., Finn, R.D., Hollich, V., Griffiths-Jones, S., Khanna, A., Marshall, M., Moxon, S., Sonnhammer, E.L., Studholme, D.J., Yeats, C. and Eddy, S.R. (2004). The Pfam protein families database. Nucleic Acids Res. 32 (Database issue): D138-D141.
Battistella, E., Souza, J.G.C., Ferreira, R.A., Vieira, R., Lemke, N. and Mombach, J.C.M. (2004). Bioinformatics: A Growing Field for Ontologies. In: Proceedings of the Workshop on Ontologies and their Applications (WONTO’2004), São Luis, MA, Brazil, pp. 93-103.
Brazma, A., Hingamp, P., Quackenbush, J., Sherlock, G., Spellman, P., Stoeckert, C., Aach, J., Ansorge, W., Ball, C.A., Causton, H.C., Gaasterland, T., Glenisson, P., Holstege, F.C., Kim, I.F., Markowitz, V., Matese, J.C., Parkinson, H., Robinson, A., Sarkans, U., Schulze-Kremer, S., Stewart, J., Taylor, R., Vilo, J. and Vingron, M. (2001). Minimum information about a microarray experiment (MIAME) - toward standards for microarray data. Nat. Genet. 29: 365-371.
Gene Ontology Consortium (2004). The Gene Ontology (GO) database and informatics resource. Nucleic Acids Res. 32: 258-261.
Goble, C.A., Stevens, R., Bechhofer, G., Ng, S., Paton, N.W., Baker, P.G., Peim, M. and Brass, A. (2001). Transparent access to multiple bioinformatics information sources. IBM Systems Journal Special Issue on Deep Computing for the Life Sciences 40: 532-552.
Gruber, T.R. (1993). Toward principles for the design of ontologies used for knowledge sharing. In: Formal Ontology in Conceptual Analysis and Knowledge Representation (Guarino, N. and Poli, R., eds.). Kluwer Academic, Deventer, The Netherlands.
Hermjakob, H., Montecchi-Palazzi, L., Bader, G., Wojcik, R., Salwinski, L., Ceol, A., Moore, S., Orchard, S., Sarkans, U., von Mering, C., Roechert, B., Poux, S., Jung, E., Mersch, H., Kersey, P., Lappe, M., Li, Y.X., Zeng, R., Rana, D., Nikolski, M., Husi, H., Brun, C., Shanker, K., Grant, S.G.N., Sander, C., Bork, P., Zhu, W.M., Pandey, A., Brazma, A., Jacq, B., Vidal, M., Sherman, D., Legrain, P., Cesareni, G., Xenarios, L., Eisenberg, D., Steipe, B., Hogue, C. and Apweiler, R. (2004). The HUPO PSI’s Molecular Interaction format - a community standard for the representation of protein interaction data. Nat. Biotechnol. 22: 177-183.
Ideker, T., Thorsson, V., Ranish, J.A., Christmas, R., Buhler, J., Eng, J.K., Bumgarner, R., Goodlett, D.R., Aebersold, R. and Hood, L. (2001). Integrated genomic and proteomic analyses of a systematically perturbed metabolic network. Science 292: 929-934.
Karp, P.D. (2000). An ontology for biological function on molecular interactions. Bioinformatics 16: 269-285.
Lemke, N., Heredia, F., Barcellos, C.K., Reis, A.N. and Mombach, J.C.M. (2004). Essentiality and damage in metabolic networks. Bioinformatics 20: 115-119.
Orchard, S., Kensey, P., Hermjakob, H. and Apweiler, R. (2003). Meeting review: The HUPO Proteomic Standard Initiative meeting: towards common standards for exchanging proteomic data. Comp. Funct. Genomics 4: 16-19.
Spellman, P.T., Miller, M., Stewart, J., Troup, C., Sarkans, U., Chervitz, S., Bernhart, D., Sherlock, G., Ball, C., Lepage, M., Swiatek, M., Marks, W.L., Goncalves, J., Markel, S., Iordan, D., Shojatalab, M., Pizarro, A., White, J., Hubley, R., Deutsch, E., Senger, M., Aronow, B.J., Robinson, A., Bassett, D., Stoeckert Jr., C.J. and Brazma, A. (2002). Design and implementation of microarray gene expression markup language (MAGE-ML). Genome Biol. 3: 461-469.
Uetz, P., Ideker, T. and Schwikowski, B. (2002). Visualization and Integration of Protein-Protein Interactions. Cold Spring Harbor Laboratory Press, Cold Spring Harbor, NY, USA, pp. 623-646.
Yeger-Lotem, E., Sattath, S., Kashtan, N., Itzkovitz, S., Milo, R., Pinter, R.Y., Alon, U. and Margalit, H. (2004). Network motifs in integrated cellular networks of transcription-regulation and protein-protein interaction. Proc. Natl. Acad. Sci. USA 101: 5934-5939.
|
|
|
|