|
- A bioinformatics analysis of alternative exon usage in human genes coding for extracellular matrix proteins
- Noboru Jo Sakabe1,2, Maria Dulcetti Vibranovski1,2 and Sandro José de Souza1
- 1Ludwig Institute for Cancer Research, São Paulo Branch, SP, Brazil
- 2Ph.D. Program, Departamento de Bioquímica, Instituto de Química da Universidade de São Paulo,
- São Paulo, SP, Brazil
- Corresponding author: S.J. Souza
- E-mail: [email protected]
- Genet. Mol. Res. 3 (4): 532-544 (2004)
- Received October 4, 2004
- Accepted December 13, 2004
- Published December 30, 2004
ABSTRACT. Alternative splicing increases protein diversity through the generation of different mRNA molecules from the same gene. Although alternative splicing seems to be a widespread phenomenon in the human transcriptome, it is possible that different subgroups of genes present different patterns, related to their biological roles. Analysis of a subgroup may enhance common features of its members that would otherwise disappear amidst a heterogeneous population. Extracellular matrix (ECM) proteins are a good set for such analyses since they are structurally and functionally related. This family of proteins is involved in a large variety of functions, probably achieved by the combinatorial use of protein domains through exon shuffling events. To determine if ECM genes have a different pattern of alternative splicing, we compared clusters of expressed sequences of ECM to all other genes regarding features related to the most frequent type of alternative splicing, alternative exon usage (AEU), such as: the number of alternative exon-intron structures per cluster, the number of AEU events per exon-intron structure, the number of exons per event, among others. Although we did not find many differences between the two sets, we observed a higher frequency of AEU events involving entire protein domains in the ECM set, a feature that could be associated with their multi-domain nature. As other subgroups or even the ECM set in different tissues could present distinct patterns of AEU, it may be premature to conclude that alternative splicing is homogeneous among groups of related genes.
Key words: Alternative splicing, Extracellular matrix, Exon skipping, Bioinformatics, Human transcriptome, Expressed sequences
INTRODUCTION
Alternative splicing is a phenomenon that generates protein diversity, either by including or excluding portions of a transcript important for the regulation of its translation, or by directly changing its coding sequence. There are mainly three types of alternative splicing: intron retention in the mature mRNA, alternative exon usage (AEU), resulting in exon skipping, and the use of cryptic splice sites that may elongate or shorten an exon (for review, see McKeown, 1992).
In the last few years, different strategies have been used to explore the diversity generated by alternative splicing at the transcriptome level (Burke et al., 1998; Gelfand et al., 1999; Mironov et al., 1999; Kan et al., 2001; Modrek and Lee, 2002). Most of these strategies are based on the use of expressed sequence tags (ESTs) to assess the repertoire of splicing variants. It is a consensus that at least half of all human genes present this phenomenon.
Although alternative splicing seems to be widespread in human and other organisms, it is possible that it does not affect all genes in the same way. If the generation of splicing events is tightly regulated, producing only specific variants of genes, one could expect to observe peculiar patterns (we call “pattern” the collection of features related to AEU that can describe how this type of alternative splicing affects a group of genes, like number of events, number of alternative exons, length of events, and other features) in different subgroups of functionally or structurally related genes. Analysis of a subgroup may be revealing by enhancing common features of its members that would otherwise disappear in a larger, more heterogeneous population.
The genes encoding extracellular matrix (ECM) proteins are an excellent set for a more detailed analysis of alternative splicing. These proteins constitute a family of typically large molecules that are involved in multiple functions, including structural roles in tissues such as bone, cartilage and skin, cell signaling, as well as promoting cell adhesion and migration. Often, ECM proteins are involved in interaction among themselves or with other proteins. The genes that encode these proteins are single copy and are composed by multiple exons. Alternative exon usage is known to be a common mechanism that generates diversity in transcripts coding for ECM proteins (reviewed by Boyd et al., 1993). Using punctual examples, these authors showed that many ECM proteins such as fibronectin, tropoelastin, collagens, and proteoglycans have their structures and functions modified by alternative splicing. For example, the isoform V120 of fibronectin differs from V95 by the presence of an extra portion of 25 amino acids in the N-terminus, which is responsible for adhesion of particular cell types as lymphocytes, monocytes and melanoma. Thus, the presence or absence of small portions of an ECM protein may lead to dramatic effects on cell physiology.
Many of the ECM proteins are structurally related: they share modules or domains that are associated with their multifunctional nature (Hohenester and Engel, 2002; Campbell, 2003). Exon shuffling played an important role in their constitution, that is, they were built by rearrangement of exons and protein domains or modules through intron recombination (Patthy, 1996; reviewed in Patthy, 1999). This phenomenon possibly accelerated their evolution and is commonly associated with the explosion of metazoan life in the Cambrian era. Although very efficient, exon shuffling generates diversity at the genetic level. Alternative splicing, on the other hand, generates diversity at the epigenetic level. Thus, exon shuffling is a means of obtaining static diversity. Alternative splicing may provide dynamic diversity to the same genes.
Using expressed sequence data, we performed a large-scale comparison of two sets of genes with respect to particular aspects related to alternative exon usage: one formed by ECM genes and another formed by all other known human genes. We did not observe differences in most of the features analyzed. However, splicing variants involving entire domains were more frequently found in the ECM set. This feature seems to be due to the short multiple domains of ECM genes, since the same pattern is observed in other genes presenting repeated domains.
MATERIAL AND METHODS
Data sources
Complete human genomic sequences (build 29) were obtained from the NCBI. Human ESTs were obtained from human dbEST (July 2002) (Boguski et al., 1993) and mRNA sequences derived from known human genes (called here full-insert cDNAs) were obtained from UniGene (release 153; Schuler et al., 1996).
Genome mapping of cDNAs
Contigs masked by NCBI were used. Pairs of genomic and transcribed sequences were defined using MEGABLAST (Zhang et al., 2000). Only pairs that aligned at least 45% of total transcribed sequence length, presenting exons with identity at least 93%, were considered.
cDNA clustering
cDNA clusters were built based on the coordinates of cDNA alignments to human genomic sequences. Two sequences with at least one exon presenting a common exon/intron boundary (± 5 bp) were grouped. When a sequence had no exon/intron boundary, it had to overlap another sequence by at least 100 bp in order to be included in the same cluster.
Alternative splicing annotation
For each cluster, exons were defined by comparing the cDNA/genome alignment using only those cDNAs that span at least two exons. Sequences were represented by a binary matrix, where each row corresponds to a sequence and each column corresponds to an exon. Exons present in a given sequence were represented by 1, if absent by 0. Thus, exon skipping could be detected by scanning each row searching for 10+1, where 0+ means at least one absent exon. This database has been used in another study made by our group (Sakabe et al., 2003).
Experimental sets
Here we use the term ECM in a broader sense, meaning basement membrane components, such as laminin, collagen and others, as well as cell-adhesion proteins as fibronectin, for example. ECM clusters were selected on the basis of a key word (Supplementary File 1 at
http://www.compbio.ludwig.org.br/~noboru/ecm) search in the annotation of full-insert cDNAs present in the clusters (404 clusters). All other clusters were partitioned to a set labeled “control” (17,151 clusters).
Selection of reference full-insert cDNA for each cluster
Reference sequences where AEU events were mapped were elected for each cluster according to the following criteria: i) should be the longest available, ideally covering the whole cluster and ii) have annotated coding sequence (CDS) coordinates starting at least 100 bp from the start of the cDNA sequence and with a 3’ end >20 bp. There are many full-insert cDNAs with the CDS annotated as starting at position 1, presenting no 5’ untranslated region (UTR). The average length of the 5’ UTR of human genes is 300 bp (Lander et al., 2001). Using only annotated CDSs starting at 100, we obtained an average length of 298 bp. For the 3’ UTR, using only those full-insert cDNAs with a 3' UTR of at least 20 bp yielded an average length of 750 bp, which is in accordance with a previous observation (770 bp; Lander et al., 2001). In the ECM set, 202 clusters presented both a CDS starting at 100 and a 3’ UTR greater than 20 bp. The number of clusters fulfilling these criteria in the control set was 8,037.
Generation of a non-redundant set of sequences
The analyses were performed with a non-redundant set of sequences of each cluster. To purge redundancy, the binary matrices of a given cluster were compared to the reference full-insert cDNA in the cluster. If the sequence presented an exclusive 10+1 or a 1 in place of 0 in the reference full-insert (but not in the first and last exons), the sequence was kept. At the end, sequences with the same exon usage patterns were grouped into one representative sequence of that exon-intron structure (see Figure 1). A real example of a multiple exon event can be found in Supplementary File 2.
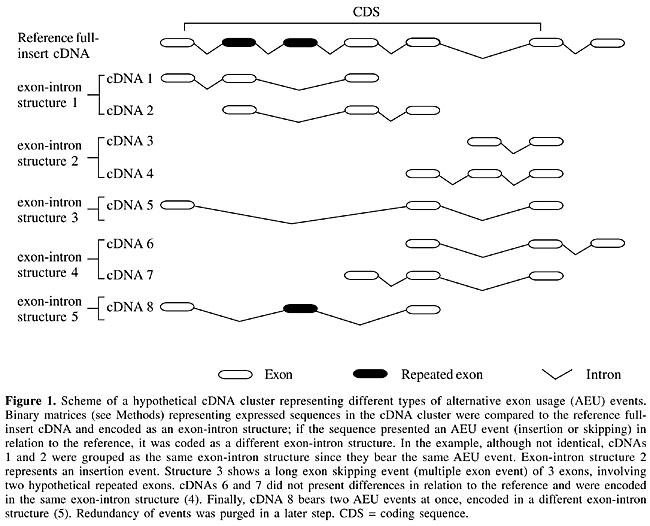
Analysis of AEU events within transcripts
To analyze the location of AEU events in a cluster (5’ UTR, 3’ UTR or CDS), we selected all clusters containing a full-insert cDNA with a known coding sequence and with more than 1 exon-intron structure. As the full-insert cDNA does not always present all the exons of the cluster, some AEU events may occur outside the reference cDNA range. As a result, 120 of 202 ECM clusters and 4,449 of 8,037 control clusters with ³2 distinct exon-intron structures composed our final data set.
An AEU event was defined as an occurrence of sequential exon skipping (10+1 in the matrices) or insertion in relation to the exons present in the reference full-insert cDNA of the cluster. The position of an AEU event was considered to be that of the ending coordinate of the last exon included in the transcript, before the skipping or insertion.
Alternative and constitutive exons
For each exon of the full-insert cDNA, the matrices of the cluster were searched for the presence of the exon. When present in all sequences, exons were marked as constitutive. An exon was considered alternative when absent and flanked by at least one exon on each side.
Analysis of protein domains affected by AEU
All the 120-amino acid sequences of the full-insert cDNAs from the ECM set and 4,449 from the control set were submitted to domain search in Pfam 12 (Bateman et al., 2002). The coordinates of domains with E-values <10-2 were converted to their cDNA positions. Domains were considered different in a given protein sequence when they presented distinct annotation and coordinates.
Simulation of the effect of random AEU events on protein domains using an artificial sequence
The artificial sequence consisted of an array of 500,000 positions, where domains were placed at each of the D positions, having length Dl. E (200) events were randomly picked having length El. For each event E, “domains” were verified if overlapping at each position D to Dl with E (random start) to El. Typical results are available as Supplementary File 3.
RESULTS
The ECM set was composed of 404 cDNA clusters containing at least one full-insert cDNA annotated with an ECM key word (see Methods). The set used for comparison was composed of all remaining clusters (17,151) and is referred to here as “control”. Among these clusters, we selected those with a full-insert cDNA that had annotated 5’ UTR, 3’ UTR and CDS (see Methods), comprising 202 ECM and 8,037 control clusters. By using a mixture of all other genes as a control set, we were able to compare the ECM to a “random” background.
Table 1 presents the features evaluated and whether there were significant differences. Data for each feature analyzed are available as Supplementary Tables at
http://www.compbio.ludwig.org.br/~noboru/ecm. Most of the features presented in Table 1 did not show statistically significant differences.
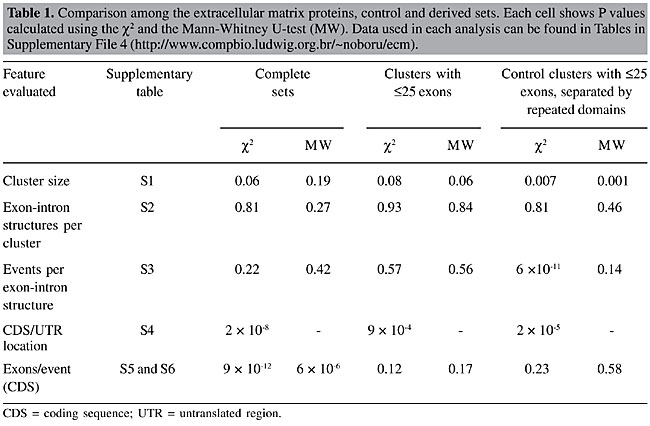
In relation to the number of exons per event, although the overall proportion of alternatively spliced exons of reference full-insert cDNAs was approximately the same in the two sets (22.3 and 20.9% in the ECM and control sets, respectively) the number of exons per AEU event (column “complete sets”) was different. Also, the location of AEU events in the CDS/UTR was different, with the ECM set presenting more events in the coding region.
The higher number of AEU events within the CDS in the ECM set could be related to a higher number of exons in this region. In fact, the ECM set has twice as many exons (22 exons/cluster versus 10 exons/cluster in the control set; Table 2). When normalized by the number of exons in the CDS, ECM clusters presented about half of the events observed for the control set (1 event/8.8 exons vs 1 event/4.8 exons in the control set; Table 2).
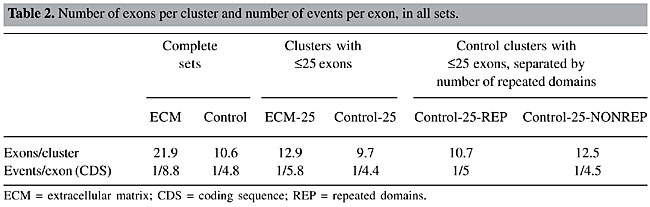
Bias caused by long genes?
Although we have no reason to believe that longer genes are different from shorter ones in relation to alternative exon usage, the former may harbor longer events. Even if only a few, they could account for the differences observed.
The distribution of the frequency of genes as a function of the number of exons is different for both sets, as shown in Figure 2. The ECM set presents a higher frequency of longer genes. Above 25 exons the frequency of “long” reference cDNAs in the ECM set drastically increases in relation to the control.

To determine a possible effect of such longer genes on the observed differences, we performed all the analyses related to AEU events previously described for both sets deprived of clusters with a reference sequence longer than 25 exons, keeping a reasonable number of clusters and a more similar distribution of the lengths of reference cDNAs. In the ECM set, such longer genes represented 16% of the clusters, whereas in the control set this fraction was 4%. The ECM set was composed of 169 clusters (92 presenting AEU) and the respective control set was composed of 7,819 clusters (4,263 presenting AEU).
There were still no considerable differences in the distributions of the features evaluated (column “clusters with £25 exons” in Table 1). Although the difference in the percentage of events in the CDS remained, the number of events per exon became similar in both sets (1/5.8 and 1/4.4 for the ECM and control sets, respectively) (Table 2).
In “long” genes of the ECM set, there were 34 of 98 (35%) non-redundant AEU events skipping ³4 exons. Sixteen skipped between 10 to 60 exons at a time. An example of an event that skips 35 exons is provided as Supplementary File 2. Therefore, the differences found for the entire ECM data set until now do not seem to be a feature of the set itself, but a bias caused by a small group of longer genes with many exons bearing very long events.
Protein domains affected by AEU
We also investigated the effect of AEU events in protein domains in the four sets (Table 3, columns “complete sets” and “clusters with £25 exons”). The ECM set presented a higher number of Pfam domains when compared to the control. ECM proteins presented more domains, not only because they are often longer, but also because the density of domains per coding exon is higher.
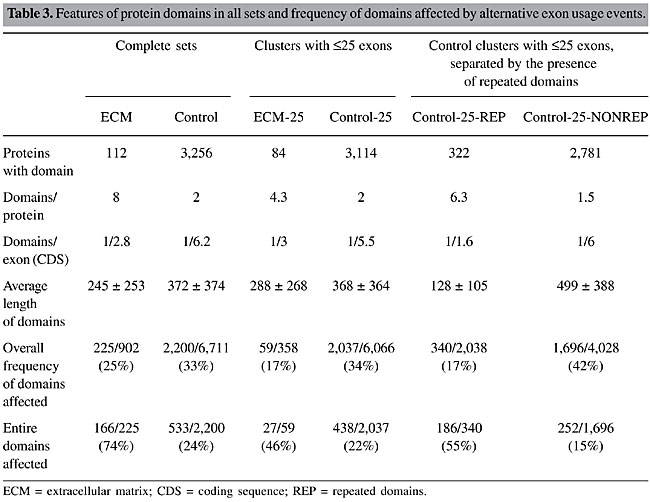
Counting the proportion of protein domains affected by AEU events revealed that the numbers were similar for both sets. While this overall frequency (domain entirely or partially skipped) was roughly similar in both sets, there were considerably more domains entirely skipped in at least one event in the ECM set (Table 3). The percentage of events that were involved in domain splicing was slightly higher in the ECM set (Table 3).
Half of the “long genes” were collagen (see Supplementary File 5 for a list of genes) that is formed by arrays of the Pfam domain “Collagen triple helix repeat (20 copies)” (PF01391, formed by 20 repetitions of GXY). Many of the other genes also presented repeated domains in arrays such as cadherin CDH23 (NM_022124), fibronectin FBN1 (NM_000138) and laminin LAMA4 (X91171). Some of the events with ³4 exons in the “long” genes of the ECM set skipped many repeated domains at once, accounting for many of the affected protein domains identified. However, the proportion of domains entirely skipped in the ECM-25 set was still higher than the proportion observed for the control-25 set (Table 3).
Analysis of genes encoding proteins presenting repeated domains
There were two main differences between the ECM and control sets in relation to protein domains: the higher density of domains per protein or per exon (Table 3) and the higher frequency of (identical) repeated domains in the ECM set (Figure 3). The different frequency of affected domains could somehow be related to such domain distribution. As the control set also contained some other multi-domain genes, such as signaling and other adhesion proteins, we subdivided the control set (without “long” genes) in those containing genes presenting £2 (control-25-NONREP, 2,781 clusters) or >2 repeated domains (control-25-REP, 332 clusters). Although the ECM-25 set did not contained only proteins with repeated domains, the comparison could reveal the impact of repeated domains on the effect of AEU on domains. All the previously compared parameters remained the same (column “control clusters with £25 exons, separated by the presence of repeated domains” in Table 1), although the distributions of cluster sizes of the two new sets were different, with the control-25-REP presenting more “smaller” clusters (Supplementary Table S1).

With regard to AEU events affecting protein domains, the control set with >2 repeated domains (control-25-REP) was similar to the ECM-25 set and different from the respective control (control-25-NONREP). This result suggests that the higher frequency of events involving entire protein domains is related to the multi-domain nature of proteins.
Although there were no differences in the number of events per exon-intron structure, exons per event and number of events in the coding region, the lengths of domains differed between the ECM and control-25-REP sets and the control-25-NONREP set, being longer in the latter (Table 3). We performed a simulation using an artificial sequence to determine the impact of longer domains on the fraction of domains affected by random AEU events. Longer domains are more frequently affected by events (there are more regions to be affected), while the fraction of domains entirely affected is lower (the event must involve a longer region; Table SI in Supplementary File 3). Therefore, the different fraction of domains affected by AEU could be explained by the different lengths of repeated and non-repeated domains.
DISCUSSION
Since the ECM set was very small, and alternative splicing variants may be a minor form (and thus have a very low representation in EST databases), we decided to use all cDNA data available to detect AEU events. This decision implies that even events reported by a single cDNA were accepted as real, possibly causing the inclusion of artifactual events. We observed that the distribution of cluster sizes (number of cDNAs per cluster) was similar in the two sets compared in this study. As the frequency of artifacts is likely to be proportional to cluster size (a poor sampling may not capture rare transcripts), we believe that any bias in one data set occurring due to such events would also be present in the other data set. Furthermore, only about one third of the exon-intron structures were represented by a single cDNA. Of these, only 2% were from “contaminated EST libraries” (as determined by Sorek and Safer, 2003). Therefore, we do not believe that such artifactual events corrupted our analysis to a significant extent.
Alternative splicing has been proposed to be responsible for generating diversity and even for the differences in complexity among organisms. In a similar way, this phenomenon could differentially contribute to diversity in different sets of functionally or structurally related genes, related to their biological roles. ECM genes are known to present alternative exon usage as a common regulatory mechanism (Boyd et al., 1993). AEU variants in ECM proteins would be frequently characterized by the presence/absence of specific protein domains. It seems reasonable to suppose that viable functional differences among variants would be achieved by incorporating (or not) the functions associated with protein domains (Pawson and Nash, 2000; Hohenester and Engel, 2002; Campbell, 2003). A factor that probably contributes to the complexity of an organism is the high connectivity of proteins involved in functions where interaction is crucial (Patthy, 2003). Alternative splicing could regulate and diversify such high connectivity of multi-domain proteins, such as those encoded by ECM genes.
The increase of functional diversity by alternative splicing of protein domains has been shown by Kriventseva et al. (2003) in a large-scale study. These authors observed that deletion of whole domains occurs at frequencies above random expectation. They also observed that even when an alternative splicing event does not remove an entire domain it affects residues important to their functions, one example being the ECM protein integrin a-7. Thus, positive selection has probably been an important factor in the evolution of alternative splicing.
It is interesting therefore that the main difference observed in our analysis was a higher frequency of events involving entire protein domains in the ECM set. Although this observation does not seem to be random (a sub-set derived from the control set containing only those proteins showing repeated domains presented the same pattern), we cannot rule out the possibility that this is due to the fact that domains are shorter in the ECM set when compared to the control. Thus, it is not possible, based on the data presented here, to define the causal agent of such observation. It seems reasonable to speculate, however, that the difference observed could be related to the multi-domain nature of proteins.
For the other features evaluated in this report, there was no difference between the ECM and the control set. This may mean that the rate and pattern of alternative splicing are similar among genes. It was previously shown that the immune and nervous systems present higher levels of alternative splicing (Modrek et al., 2001). On the other hand, Zavolan et al. (2003), in an analysis of mouse full-length cDNAs, found that there was no association between alternative splicing and categories of Gene Ontology (Ashburner et al., 2000), suggesting that diversification provided by alternative splicing is limited.
The absence of a different pattern of AEU could be interpreted as absence of specialization of alternative splicing in ECM genes, therefore originating a “random” or background pattern. Such an absence in a group of genes where diversity is fundamental has several implications. One interpretation is that the diversity provided by alternative splicing is small, or at least is not achieved by all the products generated, and a great fraction of them are likely to be unregulated. This conclusion supports the observation of Brett et al. (2002) on the limited strength of alternative splicing. They showed that the number of EST variants is not different among different organisms and therefore is unlikely to account for their different complexities.
Extrapolating our observations on ECM genes, one could hypothesize that the generation of AEU variants is not differentially regulated and thus produces equal amounts of variants in any group of genes, with similar patterns, supporting the idea of alternative splicing as a “laboratory” of new isoforms, as suggested by Gilbert (1978). New variants are most probably expressed at a low level and are thus more likely to maintain deleterious mutations since the original isoform is preserved. However, if these new variants are functionally important, they will probably be positively selected. Our group has observed signals of selection in events of intron retention (Galante et al., 2004), which gives support for the view that most splicing variants are biologically meaningful.
One cannot exclude the possibility that patterns exist among other subgroups and therefore other related genes should be investigated, with different criteria of relationship. Also, it is possible that more subtle patterns exist that could change the conclusions presented here. Another not so remote possibility is that differential patterns of alternative splicing exist when considering different tissues; since we have pooled all tissues together such differences may have disappeared.
Therefore, it may be premature to conclude that the rate and pattern of alternative splicing is homogeneous among genes of different functional categories. Most studies on this issue are preliminary or subject to various interpretations. A definitive answer will only be achieved when more analyses are performed.
ACKNOWLEDGMENTS
The authors thank Pedro Galante for helpful discussions. N.J. Sakabe and M.D. Vibranovski are supported by FAPESP fellowships.
REFERENCES
Ashburner, M., Ball, C.A., Blake, J.A., Botstein, D., Butler, H. et al. (2000). Gene ontology: tool for the unification of biology. The Gene Ontology Consortium. Nat. Genet. 25: 25-29.
Bateman, A., Birney, E., Cerruti, L., Durbin, R. and Etwiller, L. (2002). The Pfam protein families database. Nucleic Acids Res. 30: 276-280.
Boguski, M.S., Lowe, T.M. and Tolstoshev, C.M. (1993). dbEST-database for “expressed sequence tags”. Nat. Genet. 4: 332-333.
Boyd, C.D., Pierce, R.A., Schwarzbauer, J.E., Doege, K. and Sandell, L.J. (1993). Alternate exon usage is a commonly used mechanism for increasing coding diversity within genes coding for extracellular matrix proteins. Matrix 13: 457-469.
Brett, D., Pospisil, H., Valcarel, J., Reich, J. and Bork, P. (2002). Alternative splicing and genome complexity. Nat. Genet. 30: 29-30.
Burke, J., Wang, H., Hide, W. and Davison, D. (1998). Alternative gene form discovery and candidate gene selection from gene indexing projects. Genome Res. 8: 276-290.
Campbell, I.D. (2003). Modular proteins at the cell surface. Biochem. Soc. Trans. 31: 1107-1114.
Galante, P.A.F., Sakabe, N.J., Kirschbaum-Slager, N. and De Souza, S.J. (2004). Detection and evaluation of intron retention events in the human transcriptome. RNA 10: 757-765.
Gelfand, M., Dubchak, I., Dralyuk, I. and Zorn, M. (1999). ASDB: database of alternatively spliced genes. Nucleic Acids Res. 27: 301-302.
Gilbert, W. (1978). Why genes in pieces? Nature 271: 501.
Hohenester, E. and Engel, J. (2002). Domain structure and organisation in extracellular matrix proteins. Matrix Biol. 21: 115-128.
Kan, Z., Rouchka, E., Gish, W. and States, D. (2001). Gene structure prediction and alternative splicing analysis using genomically aligned ESTs. Genome Res. 11: 889-900.
Kriventseva, E.V., Koch, I., Apweiler, R., Vingron, M., Bork, P., Gelfand, M.S. and Sunyaev, S. (2003). Increase of functional diversity by alternative splicing. Trends Genet. 19: 124-128.
Lander, E.S., Linton, L.M., Birren, B., Nusbaum, C., Zody, M.C. et al. (2001). Initial sequencing and analysis of the human genome. Nature 409: 860-921.
McKeown, M. (1992). Alternative mRNA splicing. Annu. Rev. Cell Biol. 8: 133-155.
Mironov, A.A., Fickett, J.W. and Gelfand, M.S. (1999). Frequent alternative splicing of human genes. Genome Res. 9: 1288-1293.
Modrek, B. and Lee, C. (2002). A genomic view of alternative splicing. Nat. Genet. 30: 13-19.
Modrek, B., Resch, A., Grasso, C. and Lee, C. (2001). Genome-wide detection of alternative splicing in expressed sequences of human genes. Nucleic Acids Res. 29: 2850-2859.
Patthy, L. (1996). Exon shuffling and other ways of module exchange. Matrix Biol. 15: 301-310.
Patthy, L. (1999). Genome evolution and the evolution of exon-shuffling - a review. Gene 238: 103-114.
Patthy, L. (2003). Modular assembly of genes and the evolution of new functions. Genetica 118: 217-231.
Pawson, T. and Nash, P. (2000). Protein-protein interactions define specificity in signal transduction. Genes Dev. 14: 1027-1047.
Sakabe, N.J., de Souza, J.E.S., Galante, P.A.F., de Oliveira, P.S.L., Passetti, F. et al. (2003). ORESTES are enriched in rare exon usage variants affecting the encoded proteins. C. R. Biol. 326: 979-985.
Schuler, G.D., Boguski, M.S., Stewart, E.A., Stein, L.D. and Gyapay, G. (1996). A gene map of the human genome. Science 274: 540-546.
Sorek, R. and Safer, H.M. (2003). A novel algorithm for computational identification of contaminated EST libraries. Nucleic Acids Res. 31: 1067-1074.
Zavolan, M., Kondo, S., Schönbach, C., Adachi, J. and Hume, D.A. (2003). Impact of alternative initiation, splicing, and termination on the diversity of the mRNA transcripts encoded by the mouse transcriptome. Genome Res. 13: 1290-1300.
Zhang, Z., Schwartz, S., Wagner, L. and Miller, W. (2000). A greedy algorithm for aligning DNA sequences. J. Comp. Biol. 7: 203-214.
|
|
|
|